Ways to Boost Health Insurance Risk Assessment
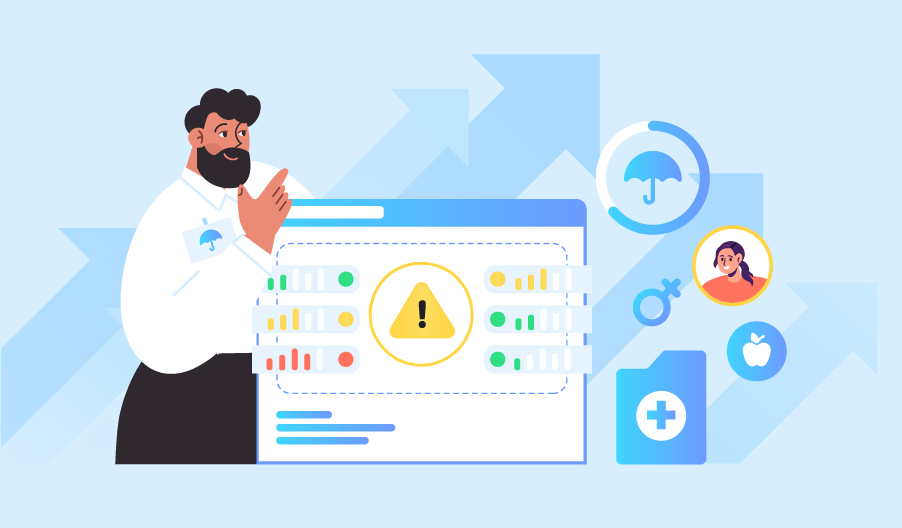
Contributors: Stacy Dubovik, Financial Technology Researcher, Alex Bekker, Head of Data Analytics Department, and Andrei Dzimchuk, Senior Solution & Integration Architect.
Digital transformation in underwriting is at the top of the health payers’ agenda to boost the speed and accuracy of risk profiling. Five out of six of my clients in health insurance believe that intelligent underwriting technology can drastically improve the way they estimate customer risks, and some plan to invest 2x more in AI-supported automation and advanced analytics over the next three years. The potential payback looks attractive: based on my observations, you may expect to produce quotes up to 12x quicker and spend up to $800 less per application.
Still, most insurers are moving cautiously, prioritizing initiatives that transform a specific part of the underwriting value chain (e.g., risk data processing or risk modeling). These granular transformations help them avoid large upfront investments and accurately measure gains in one business segment before scaling the same technology to the rest of the pipeline.
Since each of my clients comes to me with a different budget for modernization, I have developed a tier list of initiatives, from free to $1M+ in investment, to match their diverse needs and technology maturity. Let’s take a look.
Strategy #1: Using Generative AI for HRA Design
Best for: health insurers seeking to enhance risk assessment quickly and with minimal investments.
Investments: free.
Health risk assessment (HRA) remains the most popular way to learn about an applicant’s medical history, attitude toward their health, and risk-relevant habits like smoking or regularly going to the gym. The structure of an HRA questionnaire and the clarity of questions largely affect the accuracy and honesty of provided answers, as well as the depth of condition disclosure and even the applicant’s propensity to bind.
Relying on Generative AI tools to model insured personas and analyze the complexity, tone, ethicality, and relevance of risk assessment from an applicant’s perspective may help to improve questions. GenAI can also assist in drafting new questions.
- You can use any open-source tool like ChatGPT to tune the questionnaire; just make sure to remove sensitive information (full names, contact information, unique identifiers) from the prompts. Rather than specifying details about individual cases, frame your requests hypothetically or generally.
- According to McKinsey, for consistent CX, a healthy person should have to answer no more than three questions. For applicants with pre-existing conditions, the task should take a maximum of 10–15 minutes.
- Requesting a customer to e-sign the form before filling it out can double the accuracy of the declared information.
Strategy #2: Integrating With New Digital Sources of Health Risk Data
Best for: health insurers who want to get in-depth insight into customer risks while minimizing friction.
Investments: $30,000–$150,000.
Market-available APIs enable access to medical, socioeconomic, and fitness data, opening the door to improved risk assessment with minimal investments. In my recent guide to smart underwriting in health insurance, I described how to bring external data to health underwriting systems while protecting PHI under HIPAA. By proactively obtaining all available data, you get an opportunity to reduce strain on customers during application. For example, questionnaires can be reduced by over 70%.
So, what digital health risk data should you consider, and where to get it? For my clients who ask the same question, I highlight three major data categories and prioritize them for integration as follows:
- Electronic health records. In fact, 90%+ of carriers are already actively working with digital EHRs. If you are among the remaining 10%, prioritize this data category high: using EHRs as underwriting evidence can bring cost savings of $100–$800 per application.
- Socioeconomic information like the applicant’s financial posture, occupation, and lifestyle can help accurately qualify applicants for ACA programs and reveal potentially non-disclosed risk factors (dangerous hobbies or frequent visits to high-risk regions). You’ll need integration with sources like public registries, credit rating platforms, financial service providers’ systems, and social media platforms.
- Dynamic health markers coming from wearables of existing policyholders. You can get heart rate and blood pressure metrics from smart watches, blood glucose levels from smart glucometers, or inhaler usage frequency by patients with asthma and COPD from smart inhalers. Such data can be used for continuous health risk monitoring and opens the way to behavior-based insurance models. However, getting data from wearables will require higher investments. I talk about it more in Strategy #5.
Covering gaps in electronic health records with dynamic HRA questions
As it’s impossible to get consented health records of every potential client, you will still need HRA questionnaires regardless of EHR connectivity. A good practice to avoid redundancy is creating dynamic digital HRAs with questions tailored to each case based on EHR access. In such a scenario, an applicant is asked to indicate their healthcare providers from the onset. An HRA tool then checks whether the integrations with the providers’ EHRs is available and, if yes, skips general questions about the applicant’s medical history.
Strategy #3: Launching Intelligent Solutions for Health Risk Data Validation
Best for: health insurers who seek an efficient way to detect gaps in health risk data and applicant fraud.
Investments: $50,000–$200,000 for an AI component; $200,000–$400,000+ for a standalone solution.
For many of my health insurance clients identifying fraud in applications was among the most time-consuming tasks. Indeed, missing fraud at this stage poses the greatest threat to accurate risk pricing. Application forgery remains the most expensive type of insurance fraud in the U.S., costing health payers around $105 billion annually.
AI-powered prescreening tools can help underwriters quickly spot fraudulent applications, preventing deflated premiums and illegitimate access to health programs. Such solutions automatically cross-reference health risk data submitted by customers with data from trustworthy sources, such as other insurers’ and governmental databases. Intelligent algorithms instantly flag suspicious inconsistencies in the application data that may indicate schemes like not declaring inherited diseases, misrepresenting income to qualify for ACA products, and hiding existing coverage to enroll in multiple health plans.
As a practical example of a small-scale implementation in this field, my colleagues at ScienceSoft have recently delivered machine learning algorithms for medical image analysis that recognize dental insurance fraud in seconds and with 95%+ accuracy.
Large language models (LLMs) used for document validation can provide the same and higher accuracy levels across textual input checks, bringing a 100%+ increase in underwriter productivity. In one of my recent guides, I shared pragmatic ways to bring this innovative class of AI algorithms into health underwriting without compromising PHI security and compliance.
Strategy #4: Bringing Machine Learning to Health Risk Analytics
Best for: health insurers that want to streamline customer risk profiling and maximize its accuracy.
Investments: $50,000–$200,000 for an ML component, $250,000–$800,000+ for a standalone solution.
No other advanced underwriting technology has such a clear and financially rewarding effect on undewriting as machine learning (ML) for health risk prediction. According to Accenture, 80% of carriers, including health insurers, name ML-based analytics among the top game-changers for their business. 40% of large health payers already employ advanced predictive analytics, and 67% are planning to invest more in such techs in the coming years.
Analyzing massive amounts of heterogeneous applicant data, predictive ML algorithms can forecast risks much more precisely than statistical models and at a speed that is not achievable with any traditional method. Alongside regular conditions, ML can point out rare diseases like hemophilia or GSD II that incur very high medical costs and should be factored in explicitly when pricing individual and group contracts. In one inspiring ROI study, 25 insurers who employed intelligent predictive analytics for risk assessment achieved a 3–10% improvement in loss ratios, compared to the industry average, and realized around $400 million of incremental profit over five years.
Although some market-available insurance risk analysis tools use machine learning, few accommodate the specifics of health underwriting, so you’ll need to customize a lot. Customization may be particularly costly if you decide to bring unstructured feeds like wearable sensor data. Luckily, machine learning platforms like Azure Machine Learning, Amazon SageMaker, and Google’s Vertex AI enable a cost-effective rollout of custom ML analytics. From my experience with ScienceSoft, building bespoke predictive ML models may cost $50,000–$200,000, depending on the scope of risk factors, modeling scenarios, and resulting parameters.
Sample architecture of ML-powered predictive analytics by ScienceSoft. Follow the link to read a detailed description of the main solution components and data flows.
Strategy #5: Introducing Behavior-Based Health Insurance Solutions
Best for: health payers who seek to transform rather than upgrade the way they measure and price risks.
Investments: $300,000–$1,000,000+.
Some emerging health insurance models reimagine traditional risk assessment flows altogether. One prominent example is behavior-based insurance (BBI). This digital-native model relies on insureds’ dynamic health data from connected apps and wearables to access risks and dynamically recalculate premiums. According to ScienceSoft’s proprietary research, by 2035, over 60% of health payers will adopt some form of BBI. If you want to win the competitive edge in the growing market, it’s high time to move on.
So, how do you implement BBI?
Prepare to engineer proprietary software, as there are currently no market-available health BBI software products and no frameworks with prebuilt logic for automated BBI operations. The core components you’ll need to enable the model are:
- An automation module to manage health incentives and repricing rules, which can be built on top of your currently used underwriting software. Constructing safe and consistent health incentive plans assumes close collaboration with medical professionals, so the module should be accessible to your healthcare partners and provide co-creation features.
- A big data processing system to collect and systematize insureds’ health metrics produced by wearables and communicate changes to policyholders. Unless you plan to provide real-time feedback to insureds on their health and lifestyle, you can save costs by opting for batch sensor data processing.
- A price optimization engine to analyze the aggregated health metrics, auto-adjust premium amounts based on preset rules, and log behavioral changes.
- A self-service app for health insureds to overview health incentives, monitor their health, and track and pay premium fees. In my recent guide to improving digital CX in insurance, I share some tips on creating winning customer apps.
Based on my estimates, the cost of building a BBI system for health insurance starts from $300,000 and can exceed $1,000,000 for large-scale implementations. However, the potential revenue is equally high: John Hancock Life Insurance, which pioneered health BBI in the U.S., received $312 million from its BBI program in 2023 alone.
How To Drive Technology Adoption Among Underwriters
A recent report by Capgemini found that although 62% of executives recognize AI/ML’s positive impact on underwriting quality, only 43% of underwriters trust and are ready to follow intelligent suggestions. Health insurers should involve underwriters at early transformation planning stages and educate them on the new technology to drive high adoption of digital underwriting tools. From my experience, prioritizing use cases where software brings quick and apparent benefits helps secure the underwriting team’s buy-in for deeper innovations. Check this case to get an idea of how small-scale pilots increase trust in larger-scale innovation.
Underwriting digital transformation may seem daunting, but setting priorities correctly and taking a step-by-step approach will help make the task manageable, measurable, and rewarding. Whether you need to define the best strategy for your case or plan the implementation of underwriting solutions, feel free to turn to me or other consultants at ScienceSoft for assistance.
Seeking to upgrade your digital insurance operations? Our insurance IT consultants are ready to provide tailored advice to drive improvements for your specific case.